Unlocking Business Potential with Medical Datasets for Machine Learning
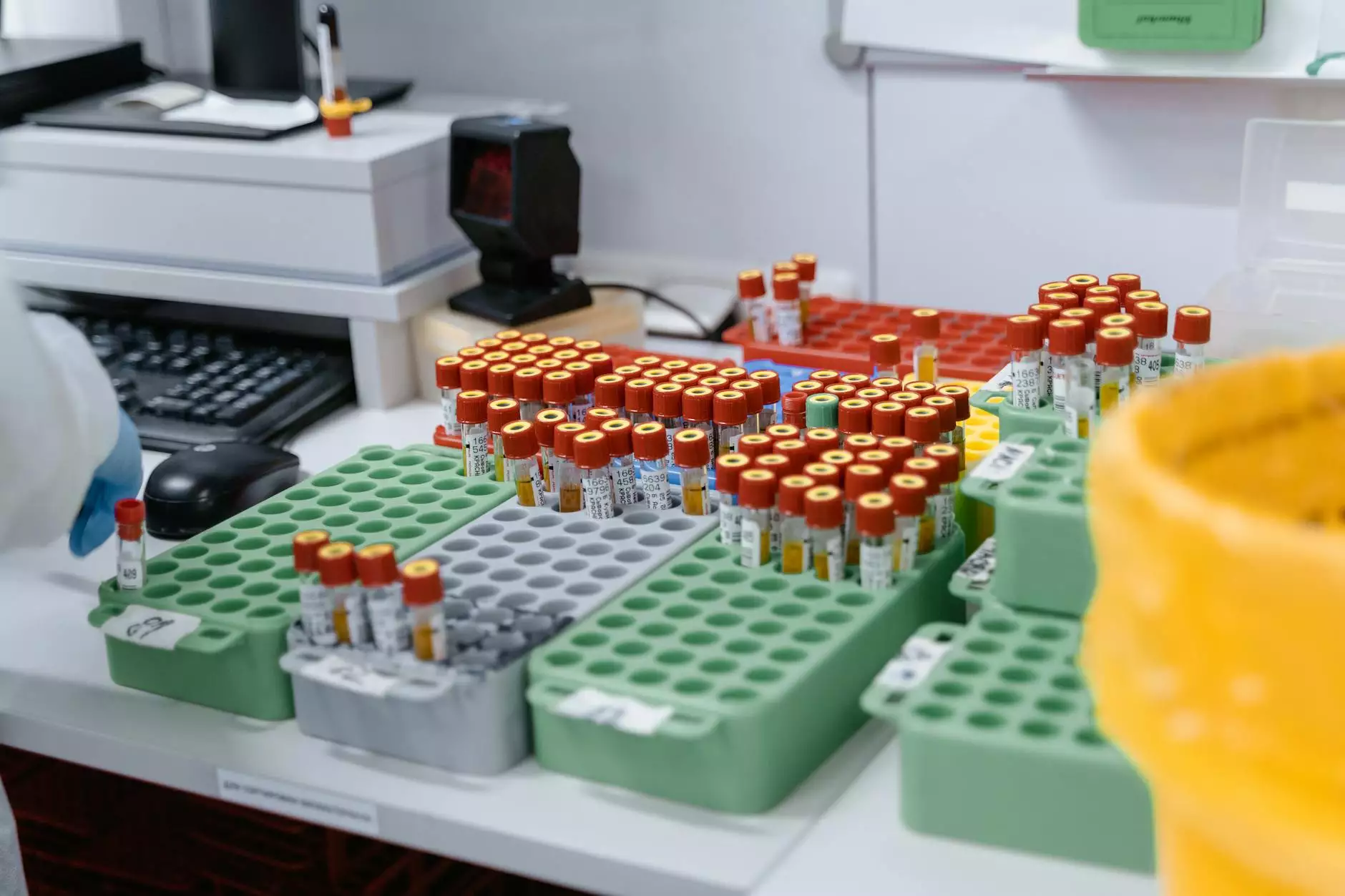
The intersection of healthcare and technology is a rapidly evolving domain. One of the critical aspects that empower businesses in this sector is the utilization of medical datasets for machine learning. This article delves into how these datasets are revolutionizing the way healthcare organizations operate, the benefits they provide, and the challenges associated with their use.
Understanding Medical Datasets
Medical datasets are collections of data derived from various healthcare activities and research. They contain invaluable information such as patient records, treatment outcomes, clinical trials, and more. These datasets can either be structured, like databases, or unstructured, like textual reports. The significance of these datasets lies in their potential to drive machine learning models, enabling enhanced decision-making and predictive analytics in healthcare.
The Importance of Data in Healthcare
Data-driven decision-making is crucial in healthcare. The integration of machine learning and medical datasets allows organizations to:
- Improve Patient Outcomes: By analyzing treatment data, businesses can identify the most effective interventions, leading to better patient care.
- Reduce Operational Costs: Efficient data management and analysis can streamline processes, thereby reducing unnecessary expenditures.
- Enhance Research Capabilities: Machine learning models can uncover insights from vast datasets, accelerating medical research and innovation.
- Facilitate Preventive Care: Predictive models can identify at-risk populations before they require critical care, allowing for timely interventions.
Business Applications of Medical Datasets for Machine Learning
Businesses in healthcare are leveraging the power of machine learning to transform various facets of their operations. Here are some key applications:
1. Predictive Analytics
Utilizing medical datasets for predictive analytics in machine learning helps in forecasting patient outcomes based on historical data. For example, healthcare providers can predict hospital readmission rates by analyzing past patient records and treatment pathways.
2. Personalized Medicine
Machine learning models trained on diverse medical datasets enable healthcare businesses to offer personalized treatment plans. By understanding individual patient data, practitioners can tailor treatments that are more effective for specific patient profiles.
3. Drug Discovery
The pharmaceutical industry is witnessing a transformation driven by machine learning. By analyzing data from clinical trials and existing medical datasets, researchers can identify potential drug candidates more efficiently, ultimately accelerating the drug development process.
4. Medical Imaging
Machine learning is significantly enhancing medical imaging analysis. Datasets derived from imaging studies enable algorithms to detect anomalies, improving diagnostic accuracy while reducing the workload on radiologists.
Challenges in Using Medical Datasets for Machine Learning
Despite the numerous benefits, there are challenges that businesses face when utilizing medical datasets for machine learning:
1. Data Privacy and Security
With the sensitive nature of healthcare data, privacy concerns are paramount. Compliance with regulations such as HIPAA in the United States and GDPR in Europe is crucial. Businesses must implement robust security measures to protect patient data while leveraging it for machine learning.
2. Data Quality and Availability
Not all medical datasets are created equal. Variability in data quality can significantly impact the performance of machine learning models. Ensuring data is accurate, complete, and representative is vital for training effective algorithms.
3. Integration Challenges
Integrating disparate data sources into a cohesive dataset for machine learning can be complex. Businesses need to invest in technologies and processes that facilitate seamless data integration while maintaining data integrity.
4. Algorithm Bias
If not properly managed, the use of biased datasets can lead to skewed machine learning models, resulting in unfair treatment outcomes. It is essential for organizations to recognize and mitigate any biases in their datasets to ensure equitable healthcare delivery.
Best Practices for Utilizing Medical Datasets in Machine Learning
To harness the full potential of medical datasets for machine learning, organizations should adopt several best practices:
- Invest in Data Governance: Establishing policies and procedures for data management is critical to ensure quality and compliance.
- Focus on Data Quality: Regularly audit and clean datasets to maintain high data quality that meets the needs of machine learning applications.
- Emphasize Collaboration: Encourage interdisciplinary collaboration among data scientists, healthcare professionals, and IT specialists to drive effective machine learning solutions.
- Implement Transparent Models: Utilize explainable AI (XAI) techniques to build trust in machine learning models, especially when deployed in clinical settings.
Future Trends in Medical Datasets and Machine Learning
As technology continues to advance, the future of medical datasets in machine learning holds promising trends:
1. Growth of Real-World Evidence
The shift towards real-world evidence will play a significant role in healthcare decisions. By analyzing data from various sources, including electronic health records (EHRs) and patient registries, machine learning can drive insights that are closer to actual patient experiences.
2. Advancements in Natural Language Processing (NLP)
NLP will continue to enhance the ability to extract meaningful information from unstructured data, such as clinical notes and patient reports, leading to richer datasets for machine learning applications.
3. Enhanced Interoperability
As healthcare data systems become more interoperable, the ability to aggregate and analyze data from diverse sources will improve, fostering better machine learning outcomes.
4. Increased Focus on Ethical AI
With growing awareness about the ethical implications of AI in healthcare, organizations will prioritize developing transparent, responsible, and ethically sound machine learning solutions.
Conclusion
The advent of medical datasets for machine learning marks a transformative stage in the healthcare sector, enabling businesses to enhance patient care, streamline operations, and drive innovation. By recognizing the potential benefits, addressing the challenges, and following best practices, organizations can harness the power of machine learning to reshape the future of healthcare. As we look ahead, the integration of these datasets will only grow, promising even greater advancements and efficiencies in the way we approach health and medicine.
medical dataset for machine learning