Labeling Training Data: The Key to Successful AI Development
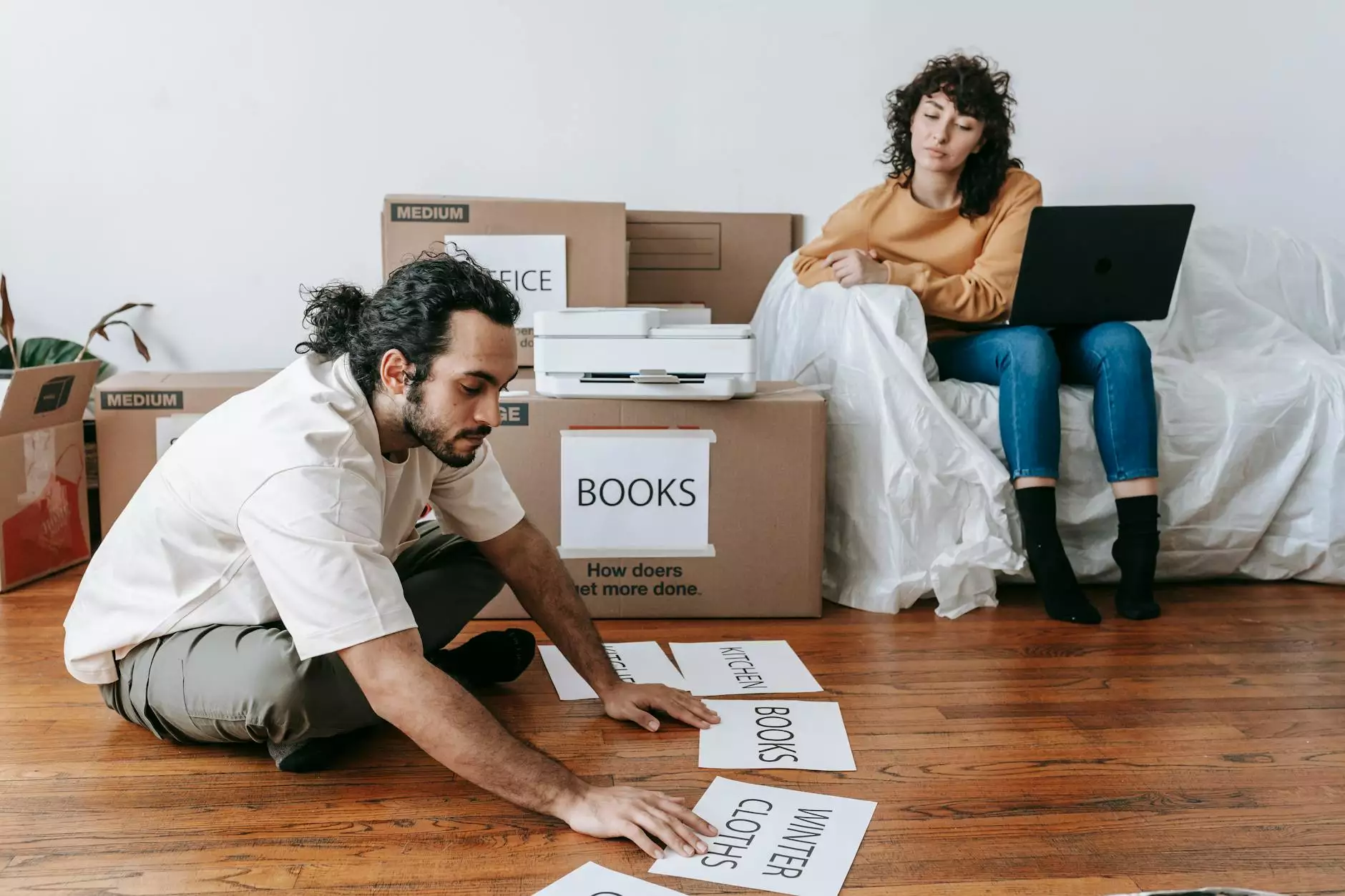
Labeling training data is crucial in the rapidly evolving field of artificial intelligence (AI) and machine learning (ML). It serves as the backbone of the data-driven ecosystem, allowing algorithms to learn from patterns and make informed predictions. In this comprehensive article, we will explore the various dimensions of labeling training data, the methodologies involved, and how data annotation tools can enhance the quality of labeled datasets, ultimately driving business growth and innovation.
What is Labeling Training Data?
Labeling training data refers to the process of categorizing and annotating raw data, making it understandable and usable for machine learning algorithms. This annotated data can be images, text, audio, or video files, and the labels indicate specific characteristics or features relevant to the learning task.
For instance, in image classification tasks, labeling might involve identifying objects within images, while in natural language processing (NLP), it could mean tagging parts of speech or sentiment in a body of text. Without properly labeled data, the performance of AI models can suffer, leading to unreliable outcomes and diminished operational efficiency.
Why is Labeling Training Data Important?
The significance of labeling training data cannot be overstated. Here are several reasons why it is a fundamental aspect of machine learning:
- Data Quality: Accurate labels improve the quality of training datasets, which is directly correlated with the performance of AI models. High-quality data leads to better predictions and insights.
- Model Accuracy: Labeled data helps machine learning models learn from historical examples, allowing them to make accurate predictions on unseen data.
- Versatility: Labeled datasets can be utilized across various domains and applications, catering to diverse business needs, from image recognition to voice assistance.
- Compliance and Ethics: As regulatory frameworks tighten, ensuring that data is properly annotated can help companies maintain compliance with data usage laws, enhancing their credibility.
Understanding Different Data Annotation Techniques
There are several methods for annotating data, each tailored to specific types of datasets and use cases. Here are some of the most commonly used techniques:
1. Image Annotation
Image annotation involves marking objects within an image, often by drawing bounding boxes, polygons, or segmentation masks. This technique is essential in applications like self-driving cars and facial recognition technologies.
2. Text Annotation
Text annotation includes tasks such as named entity recognition, sentiment analysis, and part-of-speech tagging. This type of annotation is vital for developing NLP applications, including chatbots and virtual assistants.
3. Audio Annotation
Audio annotation entails labeling audio files for tasks like speech recognition or emotion detection. It helps in training models that interpret spoken language and tone.
4. Video Annotation
Video annotation is the process of labeling video content for tasks such as activity recognition or object tracking. This is pivotal for applications in surveillance, sports analysis, and autonomous systems.
Leveraging Data Annotation Tools
To streamline the process of labeling training data, businesses are increasingly turning to advanced data annotation tools. These platforms offer automation, scalability, and flexibility that manual labeling cannot match. At KeyLabs.ai, we provide a state-of-the-art data annotation platform that empowers organizations to efficiently label diverse datasets.
Features of KeyLabs.ai Data Annotation Tool
- User-Friendly Interface: Our platform provides an intuitive interface that makes it easy for both technical and non-technical users to label data.
- Multiple Annotation Types: Support for various annotation types—including text, image, audio, and video—ensures that all your data needs can be met in one place.
- Automation and AI Integration: With our advanced AI-driven features, users can achieve higher accuracy and faster results, minimizing the human effort required.
- Collaboration and Management Tools: Our platform allows for project collaboration, enabling teams to work simultaneously and manage workloads effectively.
Best Practices for Labeling Training Data
To achieve the best results from data labeling, consider the following best practices:
1. Define Clear Guidelines
Establish clear and concise labeling guidelines to ensure consistency across the dataset. This includes defining what constitutes various labels and providing examples.
2. Employ Skilled Annotators
Utilize experienced annotators who understand the nuances of the data and can provide accurate and reliable labels.
3. Implement Quality Control Measures
Incorporate regular quality checks and reviews to catch any mislabeling early in the process. This can include random sampling and feedback loops.
4. Use Automation Wisely
While automation can significantly enhance efficiency, it is vital to monitor it closely to ensure that it doesn’t compromise quality. Balance automated and manual annotation for optimal results.
The Future of Labeling Training Data
As AI continues to advance, the methods and tools for labeling training data will also evolve. Here are some future trends to watch:
- Increased Use of AI in Annotation: Expect to see more sophisticated AI algorithms that can assist with the labeling process, making it faster and more efficient.
- Real-Time Data Annotation: As the demand for real-time AI solutions grows, so will the need for real-time data annotation tools.
- Focus on Synthetic Data: The use of synthetic data to augment real datasets will become more prevalent, necessitating new labeling techniques.
Conclusion
The role of labeling training data in AI and machine learning is undeniable. Properly labeled data is essential for creating high-performing models that can drive innovation and transformation in various industries. By leveraging advanced data annotation tools like KeyLabs.ai, businesses can enhance their data quality, improve their model accuracy, and stay ahead in a competitive landscape.
Investing in robust data annotation practices is not just a technical necessity; it is a strategic move that can significantly influence your organization's success in the AI arena. Embrace the power of labeled data and unlock your potential today.